Stat Cafe - Dr. Runmin Wang
High-dimensional inference and beyond
- Time: Monday 3/18/2024 from 11:40 AM to 12:30 PM
- Location: BLOC 448
- Pizza, cookies, and drinks provided
Description
In the first part of the talk I will introduce a general U-statistic based approach to adaptive testing for high-dimensional data.Our work extends the recent work by He et al. (2020) who proposed an adaptive test by combining U-statistics for $l_q$ norm of the parameter vector with different q’s from 2 to infinity to handle both sparse and dense alternatives. For a general parameter vector we have proved that the U-statistic for the $l_q$ norm is asymptotically normal under mild regularity conditions and such U-statistics for different q’s are still asymptotically independent, which allows us to construct adaptive test efficiently.
In the second part of the talk I will introduce a new dimension-agnostic method for change point detection. In practice, when the dimension is less than the sample size but is not small, it is often unclear whether a method that is tailored to high-dimensional data or simply a classical method that is developed and justified for low-dimensional data is preferred. In addition, the methods designed for low-dimensional data may not work well in the high-dimensional environment and vice versa. In this project we are able to construct a new inference procedure and show that the limiting null distribution for our test statistic is the same regardless of the dimensionality and the magnitude of cross-sectional dependence. Through Monte Carlo simulations and a real data illustration, we demonstrate that the finite sample results strongly corroborate the theory and suggest that the proposed test can be used as a benchmark for change-point detection of time series of low, medium, and high dimensions.
Presentation
Recording
Gallery
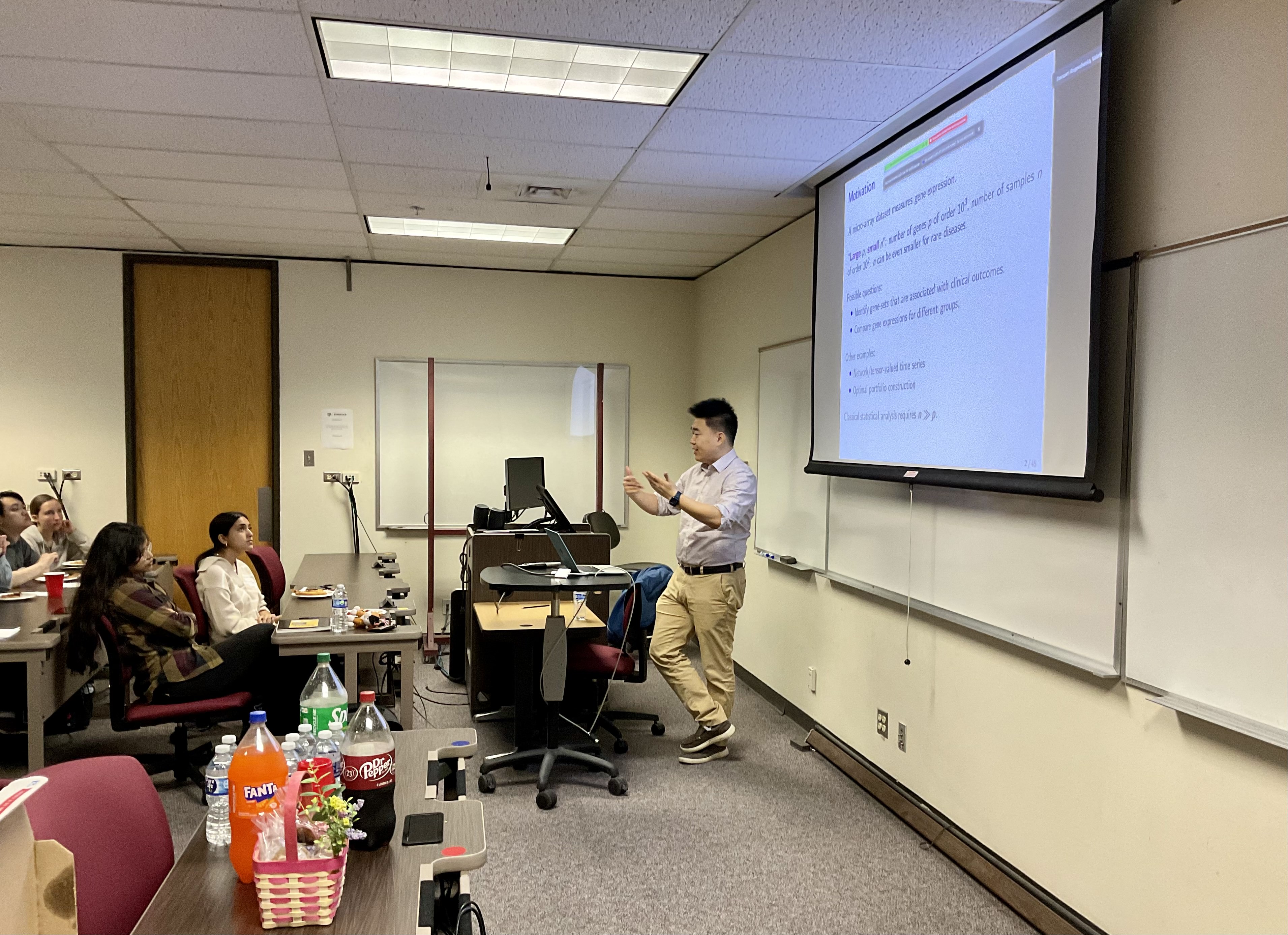
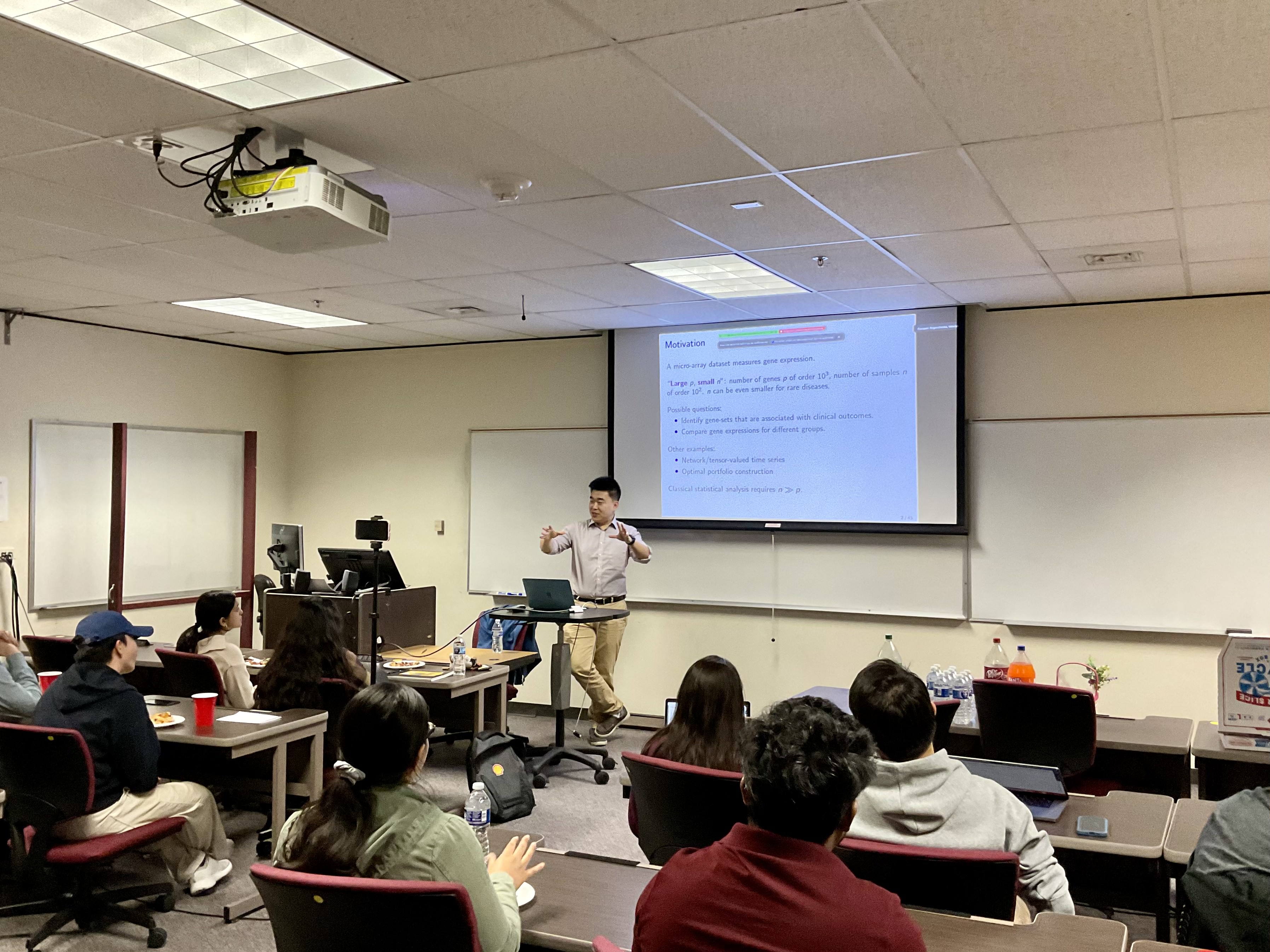
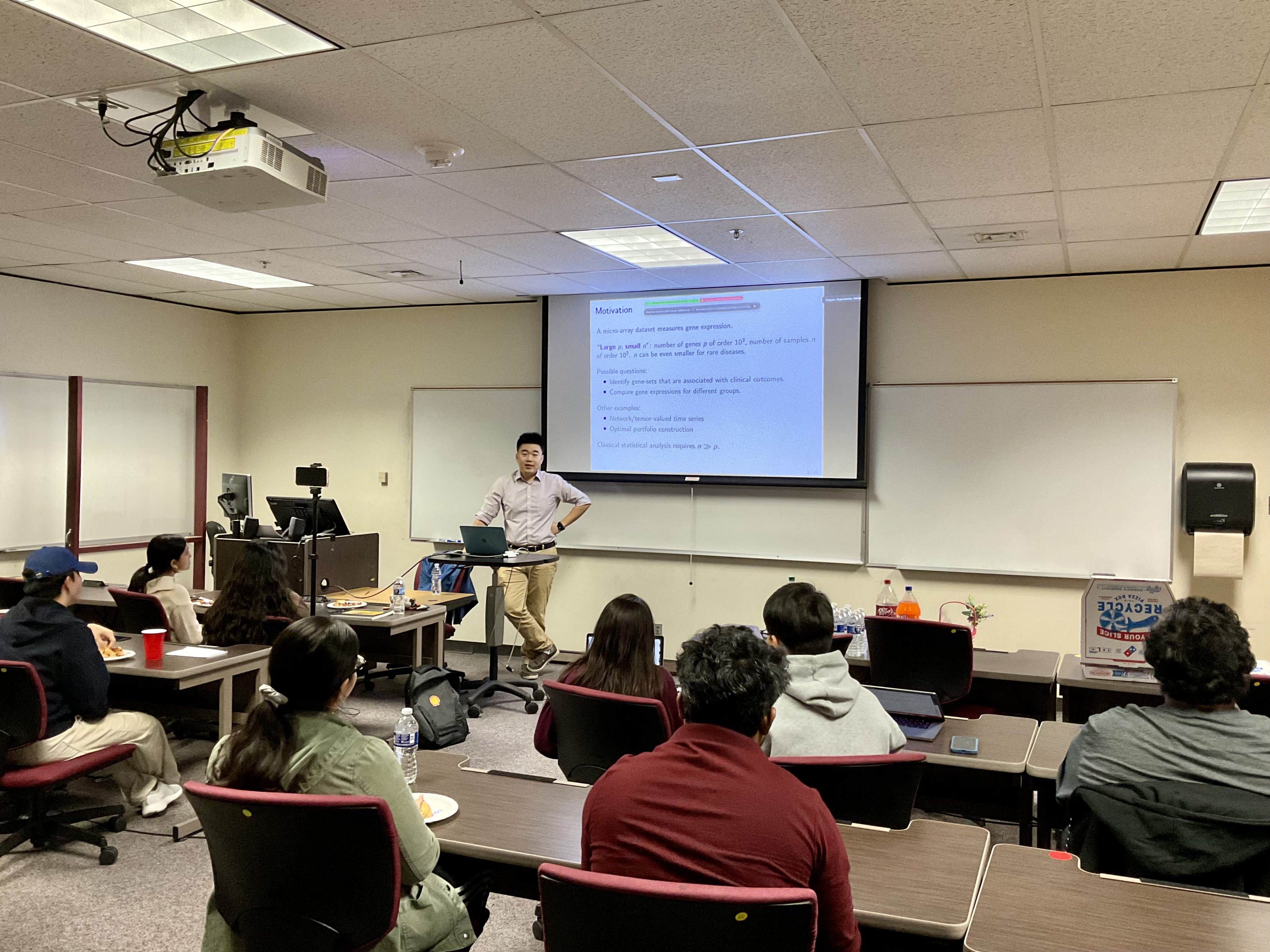
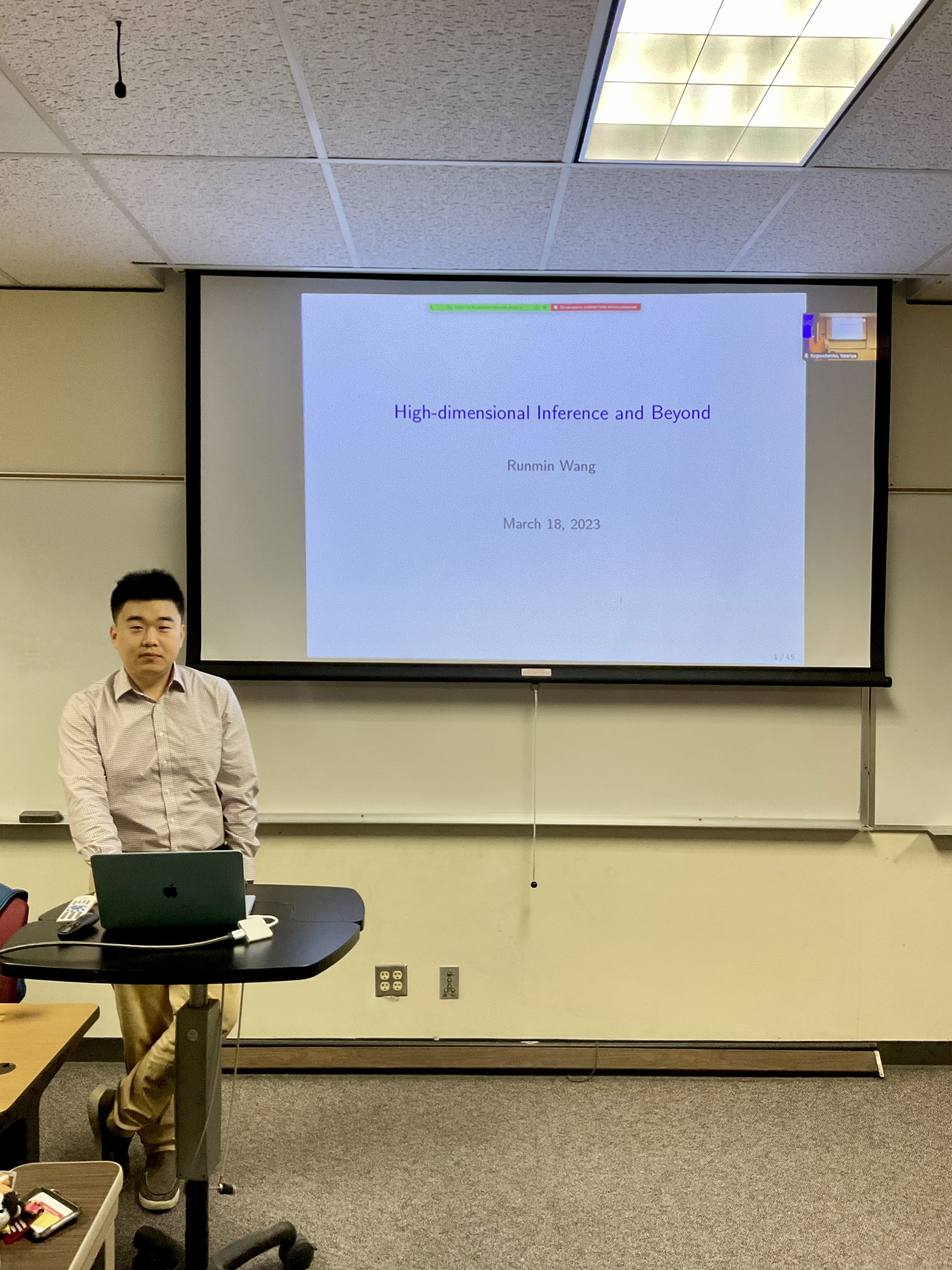
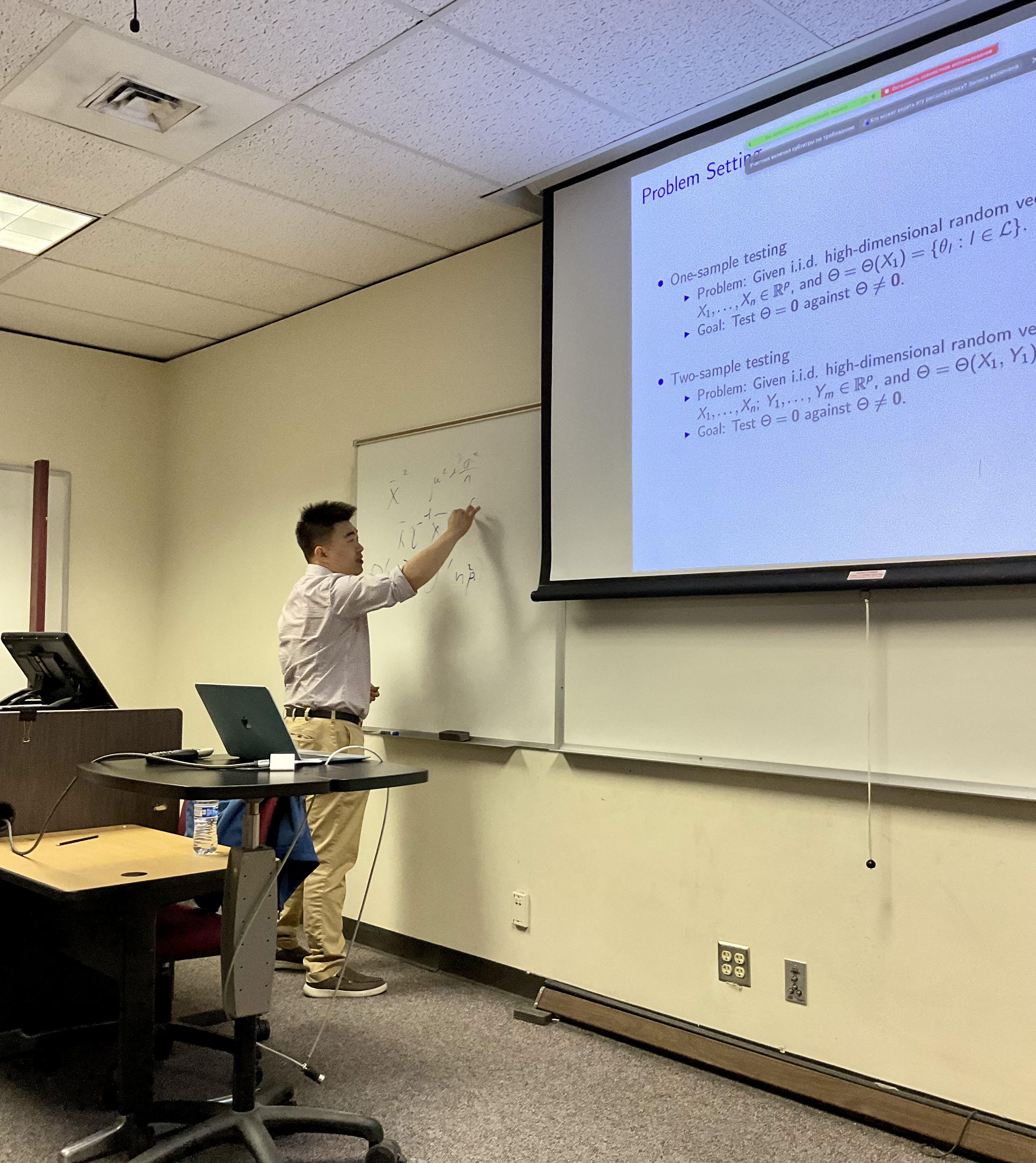